Nov 13, 2024
5 Min
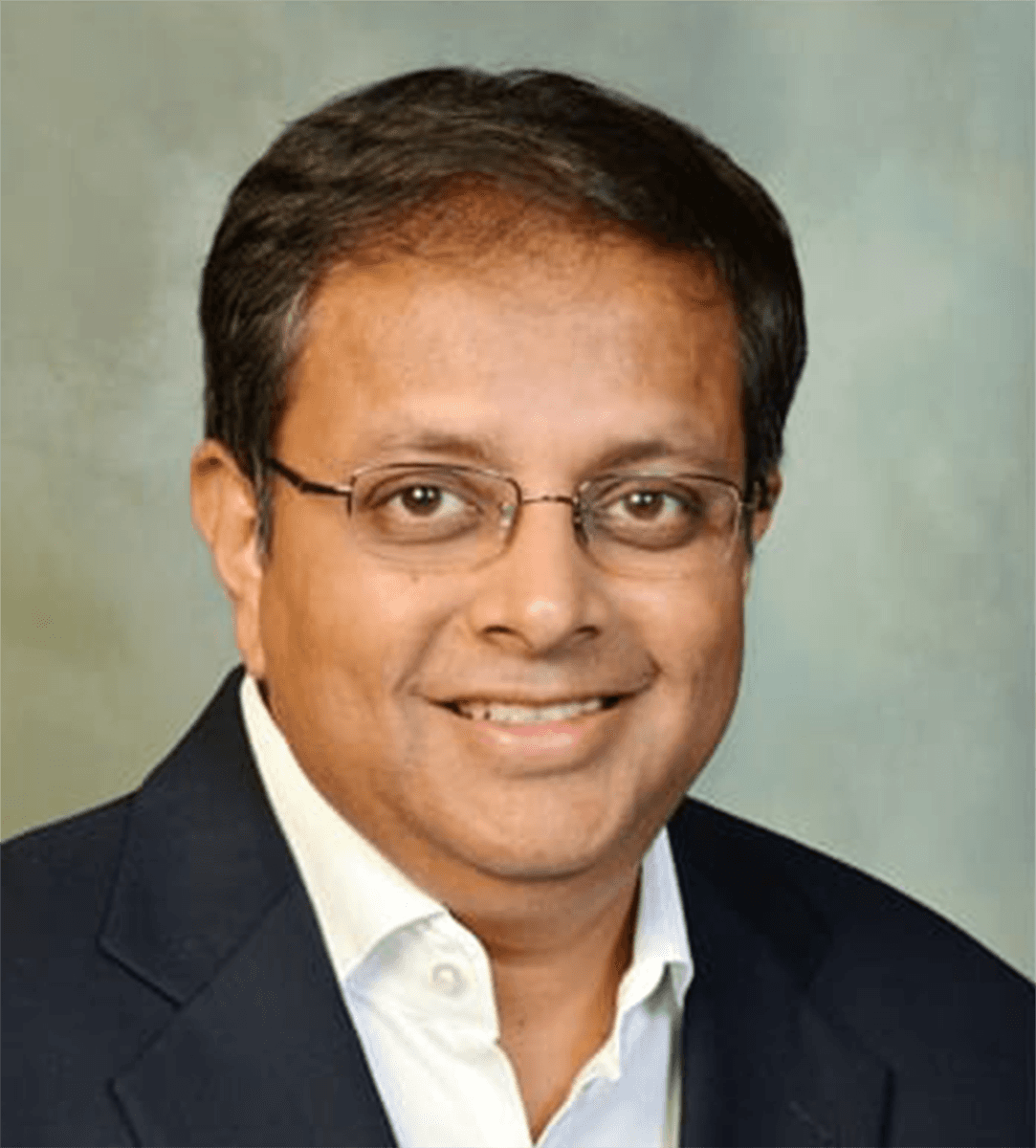
Neeraj Bhargava
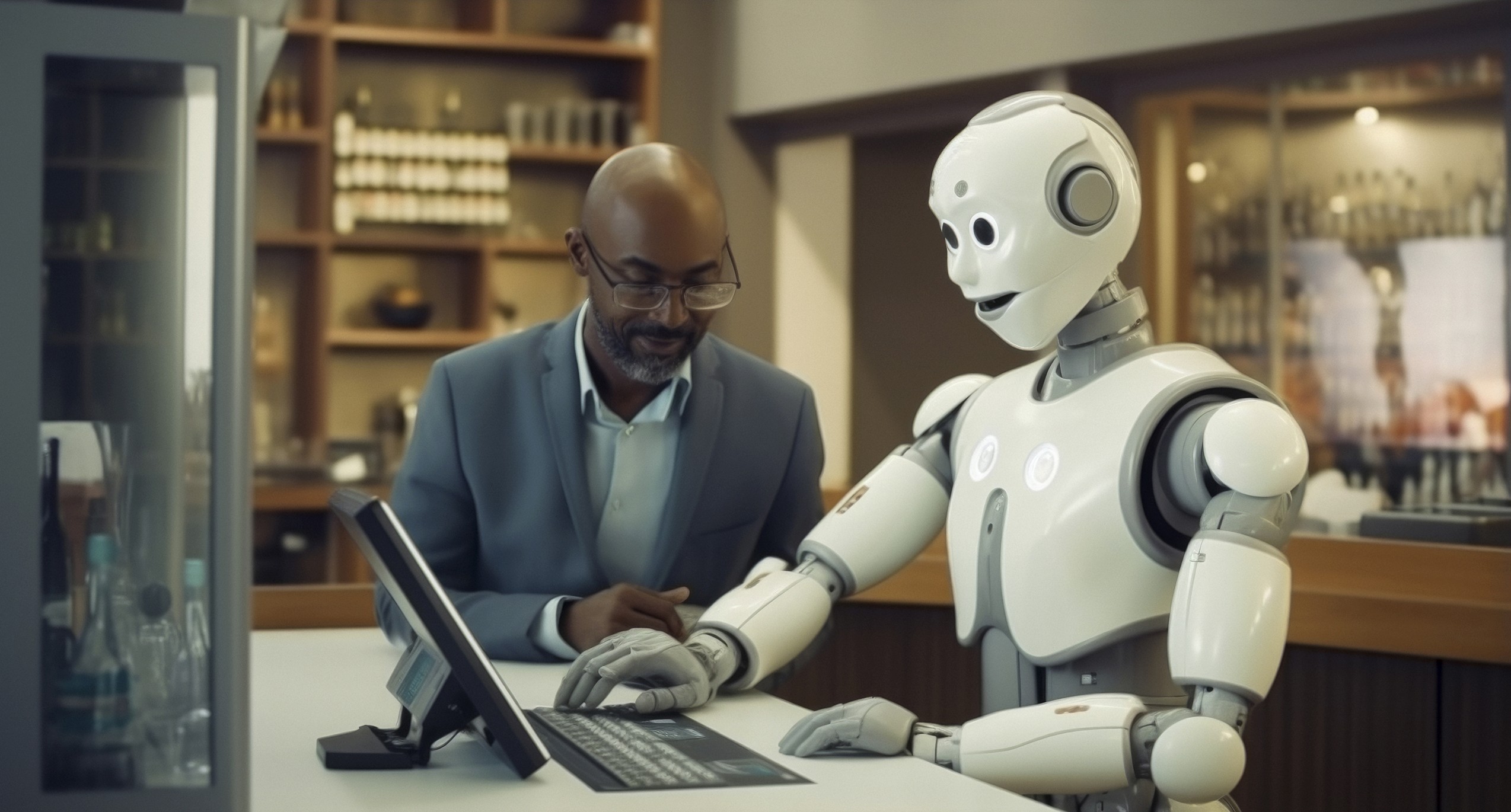
Remember Autonomous Self-Driving Cars? Google announced their initiative in 2009. It is 15 years. Except for an occasional piece of excitement about someone taking a ride in the Google campus or Elon Musk announcing a new one for China to shore up an under-pressure stock price, they are mostly non-existent. Why have they not worked and become a part of our daily lives? Because of multiple and multi-faceted challenges.
Technical challenges like sensor reliability, computer vision challenges on object recognition and lighting, Machine Learning adaptation to changing environments, cyber security issues from hacking and viruses, physical security problems from potential accidents, regulatory limitations and liability concerns, and most of all unexpected real-world surprises from construction, pedestrians and animals on the road, changing weather, etc. The list is long and diverse. The autonomous car roll-outs have therefore ended up being gradual, in controlled environments, and for limited purposes. Complete autonomy is a myth, and it is hard to visualize something like that even in the next 15 years. In Mumbai traffic, where I am right now? Nah, no chance, LOL.
The latest autonomous “things” being hyped right now are AI Agents for Enterprises. Individually and in a system. Many models barely mention a role for human beings. The reality is that AI technologies are getting more capable of handling tasks, almost autonomously. Don’t ignore the word almost. But the myth is that they can work in an organization with multiple roles and hand-offs autonomously. That belief ignores challenges similar to the ones faced by autonomous cars when they moved from a lab to the real world.
Accuracy and Hallucinations? Serious Struggle. Imagine accounts that are not 100% accurate or a customer call handled wrongly creating mishaps and liabilities or legal advice based on wrong interpretation of the law and past judgments. 1 Error will be > 1000 good occurrences.
Security and Privacy? Not sorted. LLM usage arguably is increasing security and privacy risks.
Regulatory and Liability understanding for AI? Limited. It looks like a while before AI will be allowed to approve financial and operational output and transactions. What if something goes wrong? Will insurance cover such errors? Who wants to take that risk?
Integration with existing IT systems and environment? The CIOs are just getting into figuring that out and being extra careful, rightfully so in most cases.
Customer Acceptance? Almost all our clients have told us that customers don’t want to talk to an AI. That will change as experiences get better but the issue is real now.
Employee Acceptance and Training? Huge issues here. How do SOPs change and get redesigned? Will they be killing their own jobs? What if AI makes a mistake, who is to blame? These are day-to-day questions.
AI Costs and Benefits? Experimentation is fine. What are the quantified benefits of using AI and how much does securing them cost? If there is cost or time saving, then how does that translate to value? What is the business case?
Enterprise adoption at scale will not happen till these issues are put on the forefront and addressed head on. What is in common between Autonomous Vehicles and “Autonomous” AI Agents is that they have to both work in chaos and yet be close to 100% perfect. If you do not understand that, then you are just a part of the Hype Machine and not talking about business impact in the real world. Just because something works on a few occurrences doesn’t mean that it can work flawlessly and win universal acceptance. It is important to understand that difference and solve for it. Gartner used the word “disillusion” when it comes to enterprise customer experiences with AI. One needs to take that feedback seriously and address it.
Now for the good part and how to Make AI Work. The potential benefits from AI are too huge to ignore and one cannot give up and just be overwhelmed by these challenges.
First and foremost, AI Agents can massively reduce time to complete a large number and a variety of tasks. We have the opportunity to reclaim time and use it more productively, be it for cost savings or improving lifestyles. We should look at each task or process or activity within enterprises and look for ways to reclaim time and measure the benefits.
Second, AI Agents also open up new possibilities on adding elements of multi-tasking, faster synthesis and integration of work, creating new simple to complex ‘stuff’ in seconds like emails, customer responses, a legal term sheet, a press release, a logo, a product video, etc. Plus you can have AI Agents work 24x7 and in multiple languages. Enterprise team members should be weaponized with these capabilities and well-trained to use them. Much of this magic will happen bottom-up, but only once everyone is past threshold levels of capability.
Third, the challenges mentioned above should systematically looked at as constraints that need to be overcome, often patiently. AI technologies are moving faster than how humans and organizations can absorb them. There will be more friction and roadblocks than we can imagine, especially when AI transcends from individual to group usage. Those operational obstacles should be understood and programmatically addressed.
Fourth, be crystal clear about how humans fit into your AI benefits program or you risk ignoring them as a part of your “Software Does Everything” mumbo jumbo at your own peril. While some tasks can be entirely done by AI, a process made up of several tasks will need to be human-assisted or human-managed or human-driven. Given the instability and newness of AI Agents, enterprises are very far from letting them run amok without human controls. Before Autonomous AI Agents performing anything beyond a narrow task become even a remote possibility, there will be Semi-Autonomous or Hybrid Systems, combining Humans and AI Agents in the same workflow and working in tandem.
Fifth, planning AI Agent execution should be multi-disciplinary, involving all of domain experts, AI Tech developers, and business managers. All three perspectives need to be blended to design and execute winning programs.
Sixth, be fanatical about getting close to 100% accuracy. Each point of inaccuracy or an inappropriate AI response will lead to an exponential increase in resistance to AI adoption. Customers will prefer 100% accuracy with human assistance to 95% through an autonomous AI Agent. By a hundred miles. Integrating AI interventions with the good old Six Sigma quality mindset is also not a bad idea.
Seventh, make room for even higher levels of human ingenuity in AI programs. Just as AI, we will evolve too. We will learn, improvise, jugaad (Indian term for innovative fixes), and be able to perform and compete at a higher level. Don’t lose sight of the positive loops that will emerge from AI.
Finally, get an AI Boss and let him/her lead and put this together. Directly accountable to the CEO. Without that the challenges and obstacles will overwhelmingly overpower the AI Agent initiatives. The promise will be lost even before it starts to take shape.
2024 has been a year when Hype was > Reality. 2025 is when Reality should fight back. By winning lots of small battles. AI adoption war stories will be more about guerrilla warfare than one big perfectly planned attack. The prize is huge. Get ready for it. Focus on impact from a true AI-human partnership rather than stereotypes about magical autonomous AI Agents that supposedly do everything.